Big Data Architecture
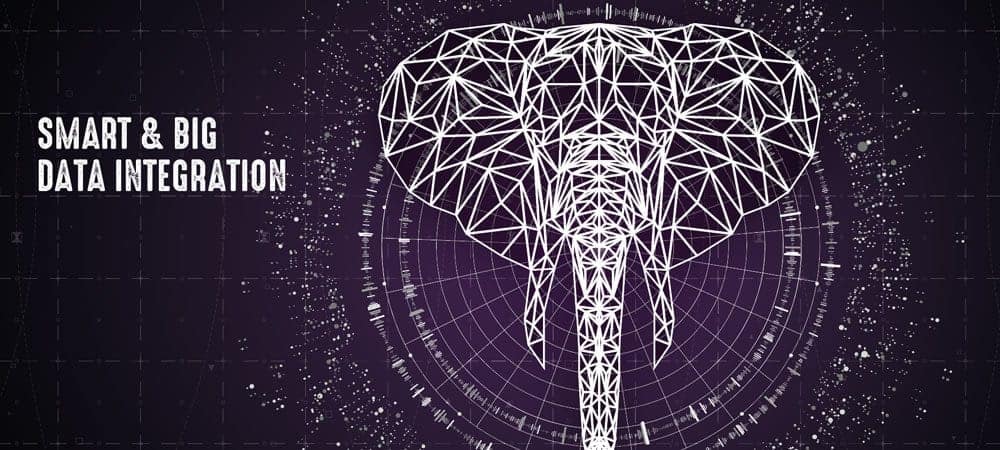
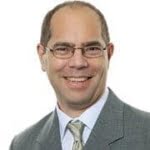
As a software architect, my goal is to achieve complicated tasks via simple solutions. The individual components of a solution each have advantages and disadvantages, the art is to combine them in such a way that in sum the advantages remain and the disadvantages cancel each other out.
For many SAP users, the first step will be to enable analytics with Big Data, that is, to find interesting information in these huge volumes of data.
But instead of building a completely new infrastructure for the users, I combine the Big Data system with the existing data warehouse.
The data scientist gets the data lake, a data area in which all the raw data is available, and a powerful tool to go with it, with which he can also process this raw data. The result of his work is new key figures that I add to the data warehouse. This has several advantages:
- The business user continues to use his usual tools for analysis, only now he has more key figures.
- The Data Scientist has access to all data, Big Data and ERP data.
- For IT, the effort is manageable.
This solution is also attractive in the context of costs vs. benefits vs. probabilities of success: By docking on to the existing, I have a reduced project scope, thus a minimized project risk and a cheaper implementation, but still fully exploit the potential benefits.
Thus, a Big Data solution consists of only two components: the data lake with the raw data and a server cluster where the data preparation takes place.
Data Lake or SAP Vora
In the past, SAP offered SAP Vora as a data lake and sells the Altiscale solution under the name Big Data Services. Basically, however, the data lake is just a large file system. If SAP sales nevertheless propose Vora, Altiscale or DataHub, the price and performance should be scrutinized very critically.
Why not just start with a local hard disk or the central file server in the first project phase? As long as there is enough space and the costs for the storage space are not too high, this is valid throughout. Copying the files is possible at any time and without any problems, so I don't block anything for the future.
Preparation with Apache Spark
For the processing of this data, most projects today use the open source framework Apache Spark. It allows programs for data processing to be written with just a few lines of code and executed in parallel in a server cluster.
There is no reason for me to reinvent the wheel here, especially since such an installation is very simple and can be done in ten minutes: download the package on a small Linux machine, extract it and start a master and a first worker via the start-all command.
Challenge: Algorithm
The technology is manageable with the above approach. Developing the algorithms for the new key figures is the difficult part: How can information be extracted from the mass data that will ultimately be reflected in the company's profit?
This is precisely where the success of a Big Data project is decided. That's why I think it makes sense to invest here, for example in the training of a data scientist.
In the following columns, I will answer the following questions, among others: Why use Apache Spark and not an ETL tool? Why do you need the data lake if the data is already in the data warehouse? Etc.