The time is ripe for artificial intelligence - are you?
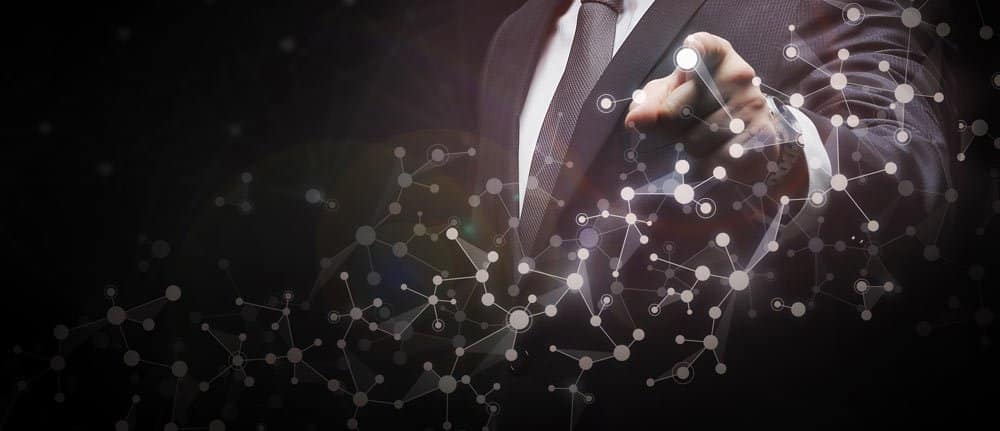
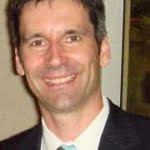
Artificial intelligence (AI) has made the transition from a pure research subject to a user technology and has become a central element of digital innovation in every industry.
The analyst firms agree on the great future of this technology - even if their estimates differ somewhat in absolute figures. The market research company Tractica, for example, forecasts that global sales of AI software will rise to around USD 60 billion by 2025.
High expectations for AI are nothing new: According to analyst firm Gartner, which measures technologies based on their perception on the market and the time it takes for them to break through, AI is already going through its third so-called hype cycle.
The starting shot was fired in the 1950s, when scientists at Dartmouth College developed programs for chess strategies. However, the projects of this initial phase were still far removed from use cases in business.
In the early 1980s, AI gave rise to expert systems (XPS). The programs helped solve complex problems and were used, for example, as computer traders in finance.
Both hype cycles were followed by disappointments, as AI failed to live up to expectations. Analysts are convinced that the current enthusiasm will not be followed by an "AI winter" for the first time and that the big breakthrough is imminent.
The reason for the renewed interest in AI since the late 1990s is based on the demonstrable success of machine learning algorithms and models that form the basis for the "intelligence" in AI.
They help machines learn tasks and then perform them autonomously - that is, they enable machines to behave adaptively or autonomously.
To be sure, some things that we humans do as a matter of course pose a major challenge for AI systems - for example, solving problems with creativity and common sense. But there are a variety of operations that are difficult for humans to learn but relatively easy for AI to master.
In industry alone, there are numerous areas of application for this and great potential for increasing productivity. Up to now, relatively simple but precisely programmed robots have reliably handled a large part of the manual operations in German production lines.
Machine learning is paving the way for scenarios in which robots complete much more complex tasks - without human intervention and without precisely predefined processes.
Machines can use historical data to learn complex relationships, for example to accurately predict from sensor data whether, when and what problems might occur with a machine. Another example is quality control, which in the past always required the judgment of a human.
Companies today have a choice of specialized IT platforms that offer sufficient computing power to process AI algorithms. These use the required data much more cost-effectively than before.
Cloud platforms and tools for data storage and processing, as well as powerful processors, are now widespread and form the basis that can meet the technical requirements of AI - in the truest sense of the word.
Thanks to the recent "Big Data" hype and the associated investments in data lakes and analytics solutions, companies have access to a wealth of information about all aspects of business processes.
For the first time since research on AI began, all the prerequisites for broad implementation of use cases in practice are in place.
Now companies need to invest and turn smart technologies and applications into economic value to gain that crucial competitive edge and remain future-proof.