Machine Learning - The Next Quantum Leap
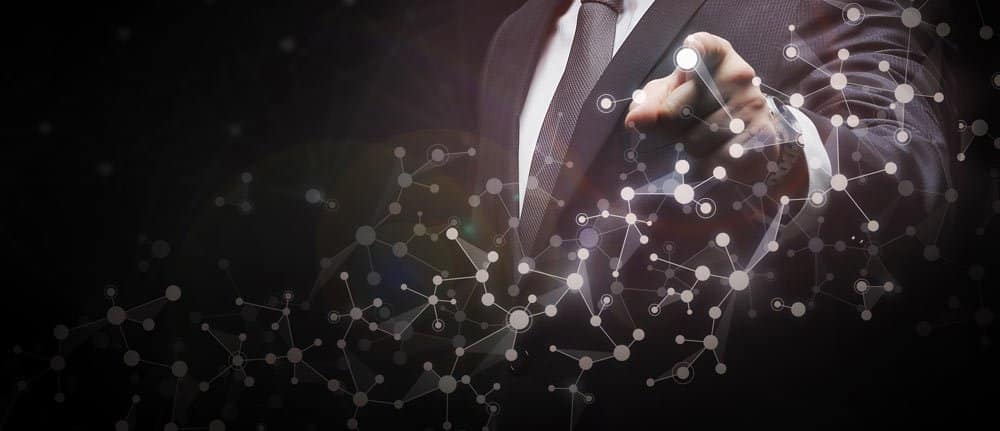
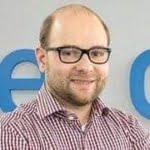
What has changed today are the technical framework conditions that make it possible to put artificial intelligence into practice:
These include greater computing power as well as affordable storage, powerful in-memory databases such as Hana, and sophisticated algorithms - but above all Big Data, a corollary of digital transformation and the basis of machine learning.
The pressure on companies is increasing: Today, they are dependent on the automation of business processes in order to withstand growing competitive and innovative pressure and to compensate for the shortage of IT specialists.
Huge data treasures are buried in systems today. Generating added value from it is already a priority in many companies today. A task that is initially far too resource-intensive.
Machine learning opens up completely new dimensions here: It is no longer humans alone, but smart algorithms that now analyze the data - and do so so quickly, extensively and intelligently that they can identify any cross-connections even within the largest volumes of data.
No human brain would be able to process so much data with comparable speed and accuracy while delivering a sound, data-based result within nanoseconds.
So far, pattern recognition has been the most commonly used variant of machine learning: establishing correlations between large amounts of data is only one subarea.
More importantly, the algorithm learns how a task can be performed. Only in this way can the software detect process deviations from the norm, for example, and make recommendations on how to transform actual processes into target processes.
By integrating machine learning components, an application can think, learn and plan quasi autonomously. This goes far beyond purely predictive data analysis.
It's about more than performing calculations according to rigid rules and triggering events. It is about agility. To achieve this and be truly predictive, a system must be easily adaptable.
After all, data, data sources, formats and processes are also constantly changing. There should be room for creativity and innovation. Insights and suggestions gained with the help of artificial intelligence should stimulate, not limit. Because in the end, real creativity, genuine lateral and different thinking, still comes from people.
When companies open up to AI, they clear the way for a small revolution. Old, role-based processes are giving way to new, more efficient and more intelligent workflows.
Machine learning can only be successful if management knowledge is combined with IT expertise and a high level of programming skill. Compared to smaller companies, large providers such as SAP have an easier time implementing self-learning systems; after all, a large part of a company's business activities runs via SAP systems.
If AI is integrated here, most of the data is available automatically - and application scenarios are obvious. Think of the allocation of payments to invoices, the selection of applicants, the evaluation of marketing ROI or forecasts of customer behavior in e-commerce.
Machine Learning also offers great potential for medium-sized companies in the Big Data environment - provided they have the necessary development capacities to integrate Machine Learning into their applications.
We at Celonis have invested a lot here and are still developing in this direction - out of the conviction that AI is the future. The impetus came not only from development itself, but also from our customers.
They not only wanted to gain insight into their own processes via classic process mining, but also wanted concrete support for making decisions: Recommendations for action on how they can further optimize individual process steps or efficiently eliminate remaining hurdles. And they wanted to know which adjustment screws were worth tackling first.