Garbage in - Garbage out
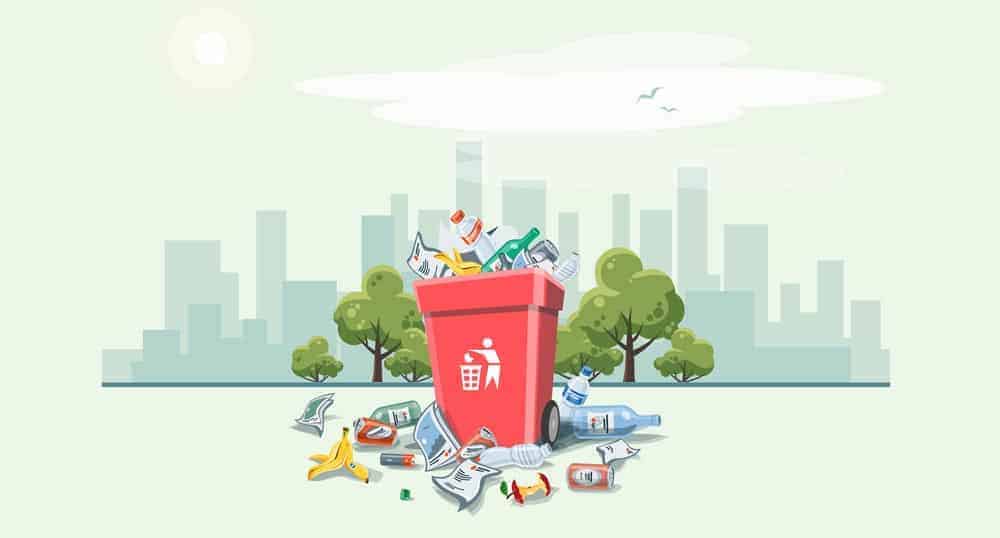
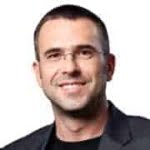
According to Lünendonk (2016), around 85 percent of companies have a master data problem. What has been known for years in the business intelligence environment is now being reflected in the digital transformation.
Companies must be prepared for the digital transformation in a process-oriented manner. This includes ensuring high master data quality. Here, new possibilities (e.g., machine learning) can now intervene directly in the process and make it possible to ensure data quality at the time it is created.
Companies must want to intervene in their business processes in this way. But those who don't will be left behind in a few years.
Two current examples
Imagine that your matching algorithm automatically writes to a candidate who is not the right one due to incomplete and not up-to-date data. How is the algorithm supposed to know what complete and up-to-date really means in relation to the candidate's data?
The costs incurred due to the unnecessary loss of time (writing mails, calling afterwards, etc.) are in fact there, but what is embarrassing is writing to the wrong candidate.
Imagine you decide on a supplier on the basis of an automatic algorithm, whose data, as in example 1, is also incomplete and not up-to-date or has even been incorrectly maintained (conditions, delivery reliability, legal requirements, etc.).
Once the order has been placed, it is difficult to cancel it. In addition to the expense of reversing the order, the credibility of the customer suffers the most.
Intervention in the business processes
In order to improve the quality of master data, the Hana platform can now be used to intervene in the business process, especially in the SAP environment. The advantage is that in Hana-based master data maintenance, the possibilities of matching algorithms can be used, which already signal to the person processing the data whether an entry is in principle correct or incorrect, or to a certain degree of probability correct or incorrect.
What means right or wrong can also be derived here in the environment of Hana technology on the basis of the already existing data. The degree of accuracy of the master data as a whole must be evaluated, defined and, if necessary, straightened out in advance.
With the data now being added, the accuracy (= consistency and completeness as well as topicality and semantics) of the data will continue to increase peu à peu and thus improve the basis for further decisions. This process uses the methodology of machine learning - learning from the accruing data.
However, the clerk is equally challenged by the process. He is the last instance to ensure the quality of the data! With machine learning, however, he is offered a tool to successfully carry out the necessary visual check.
Why Hana now?
A decisive step towards improving the quality of the master data is interactive influence through default values and/or probability statements during data entry. The empirical values from the machine learning approach must be available in real time.
An evaluation of experience values in "adjacent" data storage systems (Hadoop, etc.) will not be conducive to real-time processing of the data. The experience values (not necessarily the entire database from which these experiences were extracted) should therefore be located where they are needed at runtime - directly in the database of the operational system.
In order to use these possibilities within the SAP Hana platform, the technical licensing requirements must be met. In any case, it is an investment - but an investment that pays off.
Already today, not only can approximately five to ten percent of the total working time spent on correcting master data errors be saved, but the insights based on modern algorithms can be confidently turned into real business profit - today and ahead of competitors in the market.