Inconsistent data simply costs money
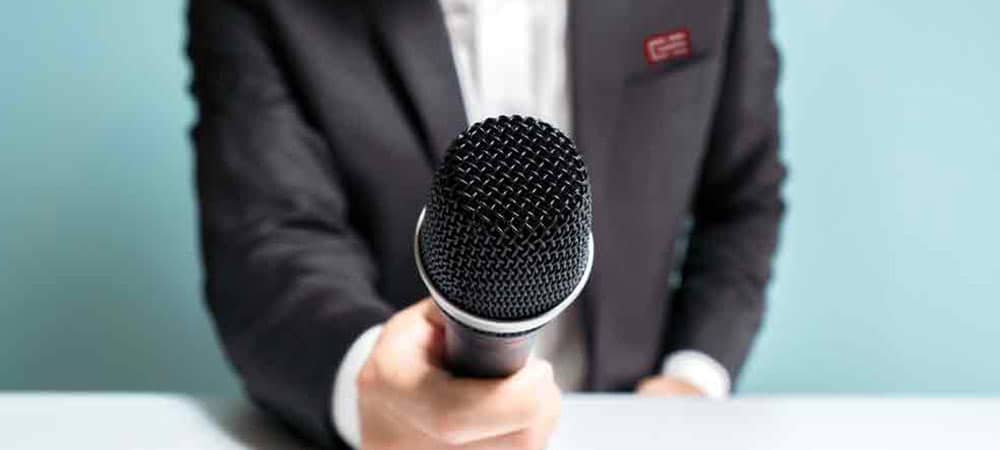

E-3: Everyone talks about data quality, but what exactly is it?
Hendrik Becker, KPMG: Data quality means getting the right answer to every question. This requires data to be constantly checked for errors, redundancy and usability. In addition to avoiding errors and gaps, it is also important to make data available to different recipients in a standardized way and to make it as easy to use as possible.
E-3: What parameters can be used to measure data quality?
Becker: Data quality can be assessed on the basis of characteristics. These characteristics include intrinsic data quality - for example credibility, accuracy, objectivity, reputation -, contextual data quality - for example added value, relevance, timeliness, completeness, data volume -, representative data quality - for example interpretability, comprehensibility, consistency of presentation, conciseness - and access quality - for example availability, access security.
E-3: What influence does the advancing digitalization have?
Stefan Riess, KPMG: Digitalization has significantly changed business activities in recent years. Many forward-looking business models such as e-commerce, online banking and e-procurement have emerged. However, digitalization has also led to far-reaching changes within companies themselves.
Stronger internal and external networking, new working models and growing investment in data analytics are just a few examples of this. The basis for all these developments is data. Without it, procurement cannot manage digital supplier lists, marketing cannot plan digital campaigns and the company cannot carry out data analyses or digitalize processes. Due to this enormous influence, the quality of the data used is also of great importance.
E-3: What does all this have to do with master data?
Becker: Whether digitized business processes in purchasing, production or sales - master data is relevant for all areas of a company. Effective master data management is the basis for digitalization initiatives in every organization. Good master data quality is not an end in itself, but enables the company to operate more efficiently and use resources more effectively.
E-3: What are the typical problems caused by poor data quality?
Riess: Inconsistent data simply costs money. If, for example, a customer or supplier exists multiple times in the system and different conditions are stored, it is easy to imagine the consequences. Poor data quality also ties up internal resources and slows down processes.
Implausible data has to be regularly rechecked, and this check often involves several departments, such as Sales, Procurement, Finance and IT, and yet final clarification is often not possible. Unreliable data sources can also lead to incorrect management decisions or market assessments and thus to a loss of market share. Finally, inadequate data quality poses an increased compliance risk due to inadequate fulfillment of regulatory requirements or insufficient transparency and traceability of operational processes.
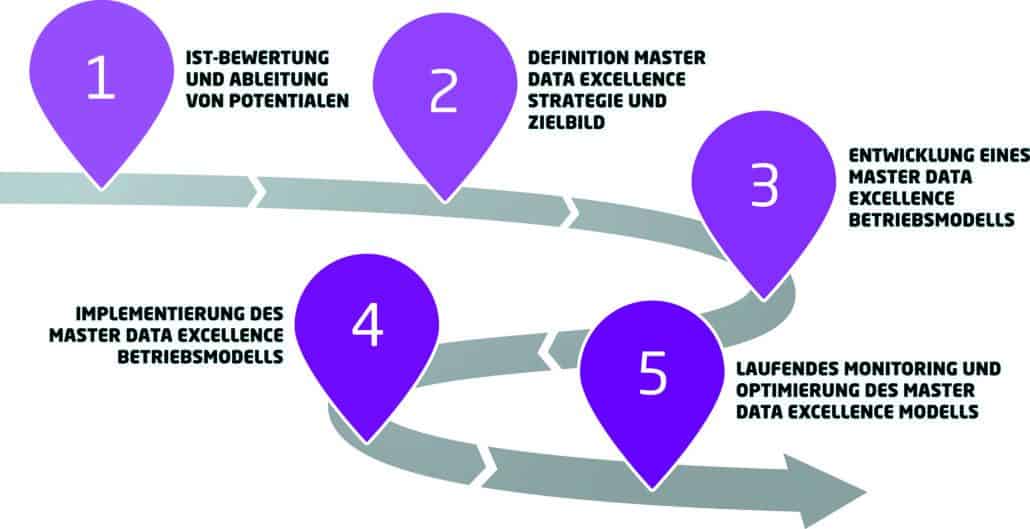
E-3: In your opinion, what are the key benefits of high data quality?
Becker: High data quality ensures faster data provision for the business units through automated workflows and information flows. Companies have reliable operational processes and a secure basis for business decisions.
By harmonizing processes and interfaces, manual and subsequent error corrections in master data records can be reduced in the long term. This also applies to bilateral coordination efforts in the context of internal and external data transfer. Last but not least, high data quality is an important prerequisite for successful digitalization initiatives.
E-3: What are the reasons for inadequate data quality?
Becker: The problem begins with the fact that there is often no transparency regarding the actual data quality. There are numerous reasons for inadequate data quality. On the one hand, the volume of data records continues to grow - for example, a product in the food industry has up to 450 attributes, such as ingredients, allergens, price recommendations and logistics information - while at the same time the number of sources and areas of responsibility for data is constantly increasing.
On the other hand, this leads to departments with divergent interests in the same data objects, too many "decision-makers" are involved and there are "perceived" veto rights in the data entry process. The authorization concept is either incomplete or does not even exist, there is a lack of clear responsibilities and escalation levels. Manual data maintenance and manual data exchange lead to inconsistent, incorrect or insufficient information.
E-3: What requirements must be met in order to establish high data quality?
Riess: First of all, it must be clear that data quality is not a purely technical problem, but above all an organizational and procedural one. Due to the cross-divisional and cross-system nature of data, an overarching and transparent responsibility for data quality is required, for example in the form of data governance.
Clear governance structures with defined roles and responsibilities as well as an escalation function in data management are essential for the efficient generation and use of data by different stakeholders. Effective, "lived" data management processes are also required, preferably separate from operational processes and technically supported by workflows or MDM tools, for example.
Finally, data quality can be flanked by a one-off data clean-sing, possibly supported by a tool. In the long term, however, it must be tackled organizationally.
E-3: Where should companies start with a master data initiative?
Becker: We recommend carrying out a maturity analysis to analyze the current situation. With the help of standardized questionnaires and workshops, those responsible can first gain an overview of the status quo and identify areas for improvement. This inventory can be combined with a system-supported data quality check, which enables benchmarking.
E-3: What contribution can software solutions make to master data management?
Becker: In master data maintenance, the right balance between complexity and efficiency is crucial. Processes for creating, changing and deactivating master data should be as simple and streamlined as possible, but as complex as necessary in order to ensure appropriate data quality. MDM tools can support this in a variety of ways.
E-3: What are some examples of solutions for better data quality?
Riess: Centralization, standardization and external verification services are the way to improve data quality in order to meet internal and external master data requirements. One example of product master data is the introduction of a centralized and automated product master. This significantly reduces manual and subsequent resource expenditure for maintaining product master data. The result: the central provision of different data from multiple and often global sources and different areas of responsibility.
E-3: What are the benefits of master data management in figures?
Becker: The results of an example project carried out by KPMG in Germany in 2020 can be used as a guide. According to this, the company involved had an improved data model after 68% of incorrect, outdated and duplicate data entries were corrected.
The efficiency of the data management process was increased by 50%, while the number of people involved in the master data process was reduced from more than 1,000 to just 50. In addition, firmly established data governance ensures data and process quality in the long term.
E-3: Data quality is not a static state. How can data quality be measured and ensured in the long term?
Riess: First of all - data quality cannot be measured one-dimensionally. Here, for example, it makes sense to use a multidimensional key figure tree - similar to (financial) controlling instruments. It first considers the characteristics of data quality and breaks them down into individual key figures, including the number of duplicates, the number of inconsistencies and the number of data fields that are not filled.
This information is linked multidimensionally with further information for root cause analysis. Other dimensions include the business unit in which the error occurred, the affected data object, the system or the process in which the data is created or used. Consideration should also be given to setting up a central master data management system.
An information map that depicts the data landscape and storage locations creates transparency. With modern data management systems, regular data and process quality analyses are pre-programmed. They can be linked to existing business intelligence systems and thus become an integral part of corporate controlling.
E-3: Thank you for the interview.
