Going Beyond Chat with AI, Hybrid Cloud, and SAP
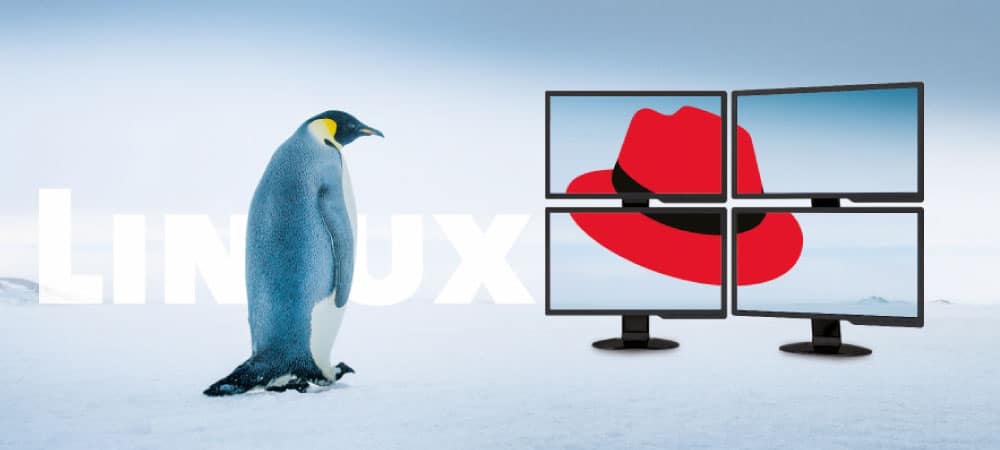
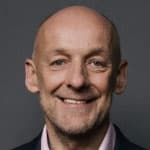
AI and ML models present several challenges and require specific frameworks and tools. This is where an established, open hybrid cloud platform and an ecosystem toolchain can also provide support.
Not least because of the hype surrounding ChatGPT and LLM (Large Language Model), AI and ML are becoming increasingly relevant for many companies, including SAP users. SAP itself is increasingly embracing AI, as evidenced by the announcement of new digital assistants based on generative AI.
However, the range of applications for AI in the SAP context goes far beyond the possibilities of a voice assistant. For example, AI is increasingly being used to analyze master data, optimize production processes and supply chains, and for quality control and even for accelerating S/4 migrations. Many companies are also increasingly developing and training models with SAP data, which they then deploy and operate in a variety of production environments, such as factory and edge scenarios.
An ideal MLOps foundation for model development, model serving and monitoring, lifecycle management, and data science pipelines across an enterprise, in the context of SAP and non-SAP data sources, is an open hybrid cloud platform. First, it provides users with access to certified AI/ML partners as part of an ecosystem. Enterprises can get complete solutions for developing, deploying, and managing ML models, making it faster and easier to deploy AI-powered intelligent applications. Privacy and secure AI operations is required to mitigate risk of exposing data and to prevent GDPR violations with personal data within training and data sets.
With Red Hat OpenShift AI, users have access to such an open source MLOps platform as a managed service and as a traditional software product, both in the cloud and on-premises. Based on a cloud-native runtime environment, it supports AI integrations in hybrid, on-premises, and edge environments, as well as different customer and application requirements. This flexibility is a major advantage when it comes to AI. On the one hand, companies can develop and train AI models with confidential data in their own data center and then run them in applications and in the cloud in a controlled manner. On the other hand, it is also possible to use the cloud to develop and train AI models, for example using anonymized or synthetic test data, and then integrate the models into an on-premises application or at the edge.
There are many benefits to using AI, especially when it comes to optimizing a supply chain or saving resources to meet sustainability goals. But an area such as automation can also benefit significantly. For example, a company can use AI to train automation on its own infrastructure. This allows new custom use cases to be developed more quickly. Red Hat is also increasing its focus on AI in automation, as evidenced by the solution Red Hat Ansible Lightspeed with the IBM Watsonx Code Assistant for AI-driven IT automation. It is aimed at the AI-generated creation of playbooks. This means it produces syntactically correct code based on AI-generated recommendations that are tailored to your IT landscape, based on customer’s existings Ansible automation content. Even complex, cross-silo automation scenarios can be implemented faster.
There is no question that the use of AI/ML techniques will increase across the board. However, RAG integration and initial deployment of AI foundation models puts a strain on an organization’s infrastructure and requires specialized platforms, frameworks, and tools, even before model serving, tuning, and management. With Red Hat OpenShift AI, Red Hat provides a consistent, scalable foundation for IT operations and a partner ecosystem for data scientists and developers to make AI innovation easier and faster for SAP users.
