Analysis and reporting systems in the cloud: ...and action!
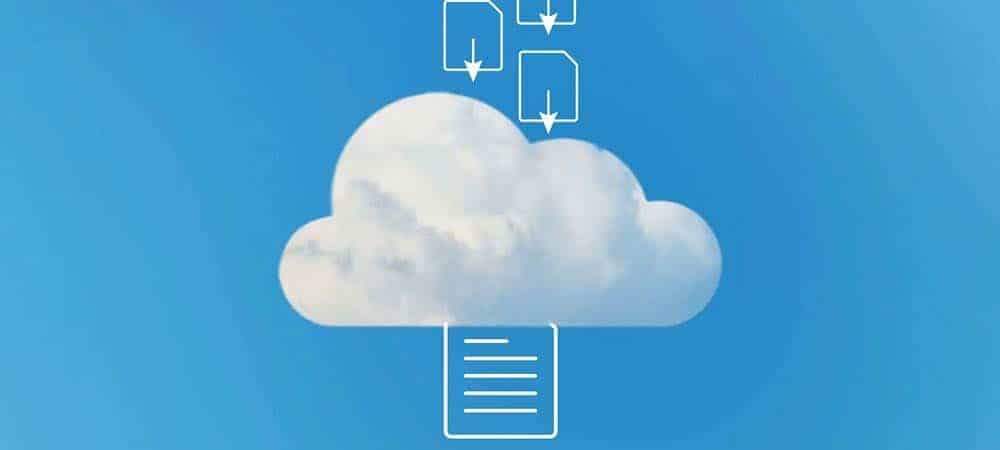
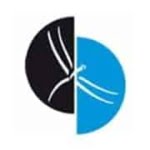
The digital transformation generates a whole jumble of data. Turning this into truly usable information is usually the task of complex and performance-intensive analysis and reporting methods developed by data scientists. However, there is often a lack of suitable systems. The cloud scenarios from Libelle AG provide a remedy.
It's like being on a movie set before the call to "...and action!" rings out. When highly paid stars are on location playing their part in the success, everything has to be perfectly prepared. It's annoying when something goes wrong right then: Faulty or only partially constructed sets, an outdated script, missing lighting bridges, weak make-up artists, too few cameras, overloaded catering. Or the set is occupied by extras scenes or visitor tours.
In many companies, situations like this are repeated regularly: large analyses in even larger data pools are due at the end of the period or as part of specific projects. The analysis results are intended to set the course for the future. Nothing should be changed during these periods, the associated systems should be reserved for specialists only, and the general business process slows down. And all systems are too slow anyway.
Other use cases also have similarly disruptive side effects: comprehensive predictions of customer behavior, complex technical calculations with different influencing parameters, analyses of customer loyalty, retention management, early risk detection, research analyses. In all these cases, performance-intensive analyses are performed on large data pools. The fact that the use of personal or other sensitive data in some areas is in the gray area of the GDPR is also often ignored.
On the other hand, the systems that departments want for this are rarely available: with up-to-date, GDPR-compliant data and powerful computing power. Performing a "quick" customized analysis interrupts the workflow permanently.
In addition to purely technical requirements, there are three main challenges:
Challenge no. 1: Performance-intensive data analysis = long processing time, high system costs
On which systems can such data analyses actually be carried out efficiently? Depending on the size and complexity of the data structures, runtime or system performance is required to reduce runtimes. In many cases, productive systems are not suitable for this - the performance requirements of complex analyses slow down actual operations.
If these are not an option, there is a call for additional systems. This is often a commercial challenge because they are seen as an investment in "stationary hardware".
Challenge no. 2: Timeliness of the relevant data
If the decision is nevertheless made "in favor of an independent analysis system", the question arises: How can it be ensured that these systems provide up-to-date data at the right time? Depending on the applications and data structures, data synchronization entails extensive manual effort. Especially in complex SAP environments, the keyword "system copy" often adds several days to the work tasks.
Challenge no. 3: handling sensitive and personal data
Once all the relevant information is available, the next issue arises: Data watchdogs are paying more and more attention to ensuring that personal data is not used for profiling without the consent of those affected. The GDPR has tightened the regulations and potential penalties. Ultimately, this raises the question: should data analysis be avoided or should data be neutralized, even if this compromises the professional significance of the data?
The "Report on Cloud" approach offers a solution to these challenges
Even if some decision-makers no longer want to hear it: cloud systems are a solution. It's not about moving productive systems to the cloud, but about hybrid cloud approaches: Productive systems on-premises, analysis systems in the cloud.
These are dedicated systems that are available exclusively for analysis and reporting purposes. The advantage: needs-based provision and billing of resources. Cloud-based analysis and reporting systems only appear in terms of costs in the time windows in which analyses are actually running, with exactly the resources (especially performance and RAM) that are used: no investment in hardware, which usually only runs at low capacity and is too weak for the purpose of analysis at some point. At the same time, the user group for these analysis systems can be severely restricted. These environments can be regularly provided with fresh and anonymized but logically consistent data that meets both GDPR and business requirements.
Setting up and operating such systems is easier than many companies still think. Providers such as Libelle and its cloud partners offer support with best practices:
The technical planning: the script.
It always starts with an idea, which leads to an initial script that is adapted by various parties to create a well-rounded story for everyone involved.
During the technical planning phase, consultants work together with the specialist, application and data protection managers to define the optimum operating model. This includes:
- Definition of specific requirements for the analysis system,
- Pre-selection of possible cloud providers including PoC planning,
- Decision template with regard to the final cloud platform,
- Detailed planning of the implementation and operating phase,
- Support for implementation.
The realization: preparing the sets and actors.
Once the script has been approved, the set is built. Realistic backdrops, artificial and real props. Actors are cast and familiarize themselves with the story and set.
Planning is followed by implementation. The analysis systems are set up, either on a "greenfield site" or as a migration of existing systems. Comprehensive know-how and, depending on the implementation option, software solutions such as Libelle DBShadow or Libelle SystemClone are available for this purpose.
Regular operation: ...and action!
Filming starts. Even with different subplots, the focus is on the main characters and the main plot. The lights are turned off during the breaks. And when the next shoot is due, the appropriate backdrops are set up.
Regular operation is divided into four phases:
Normal operation - The extra recordings: The analysis system is used with a "sufficient" performance profile by users for performance-neutral requests. Whether 7×24, 5×8 or on-demand can be regulated dynamically.
Performance phase - The stars at work: When large, performance-intensive analyses are started, the cloud system is expanded with a "performance" performance profile. The performance profile is reset at the end of the performance phase.
Rest phase - Break on set: During periods when there is little or no work, the power profile is turned down to "minimum" or the system is taken out of operation.
Refresh phase - Updating the scene images: With the refresh, current data is transferred to the analysis system - for SAP systems, for example, with Libelle SystemCopy, and for many non-SAP applications, for example, with Libelle DBCopy. An optimized performance profile is used, which is automatically adjusted as required. Personal and other sensitive data is anonymized on the basis of legal and technical requirements, e.g. with Libelle DataMasking, which ensures realistic and logically consistent data. Analyses therefore do not run on GDPR-relevant real data, but deliver real results.
The result: quality sci-fi instead of B-movie fantasy.
The end result is a movie that you can see the ingredients in: a good script, detailed sets, a whole selection of great scenes, perhaps alternative storylines, realistic masks, relaxed actors who enjoy their work. In time, in budget, in quality.
The regular result of the "Report on Cloud" approach:
- Current GDPR-compliant anonymized systems that are available on a permanent, regular or ad hoc basis and are only considered commercially according to real use.
- Analyses that process data in compliance with the GDPR and still deliver real results.
- Regular operation of the classic environments, without operational restrictions.
Analyzing large amounts of data is not solely dependent on data and software tools. They require complex interaction between different components - with as little disruption to IT operations as possible.
Visit us at the DSAG Annual Congress at stand G16.