Artificial intelligence in the context of master data management
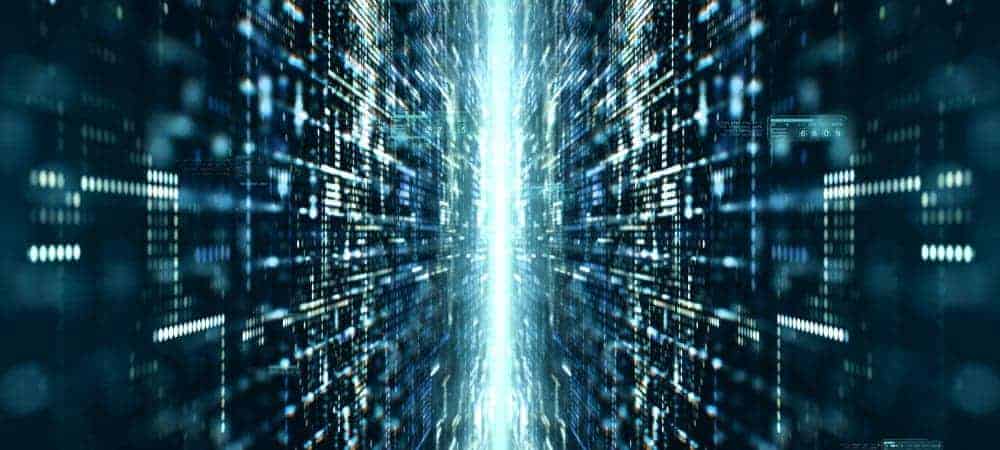
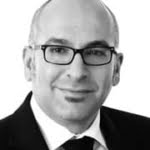
But what impact will the progress of AI have on these disciplines? And how can they be made fit for the future? Artificial intelligence (AI) in particular is said to have great disruptive potential.
However, it can be said that the understanding of AI is in a constant state of flux, as the boundary of what we believe machines and computers are capable of is constantly shifting.
Currently, science is focusing on the imitation of special human abilities such as the control of movements (robotics), the perception of the environment (machine perception) and the understanding of emotions (affective computing).
In turn, in the context of master data management, machine learning (ML) is one of the most relevant artificial intelligence capabilities.
Data consistency through AI
Data validation, based on intelligent ML systems, is a particular focus here. These systems recognize inconsistent data, for example, so-called outliers, without clear rules having been defined in advance.
Another possible use for AI in data management is master data maintenance itself. How it is done so far without the use of AI: Master data tools such as SAP MDG (Master Data Governance) can be used to define business rules that help users enter data and ensure high data quality. The biggest challenge here is that each rule to be applied must be clearly definable and derived by experienced users.
In every company, there are colleagues who check a data sheet and intuitively recognize that something is wrong. Most of the time, however, they are not able to give precise reasons why this is the case, and they are even less able to put their assumption into a defined rule.
It is precisely this ability that turns ordinary users into data experts. With the help of AI, a system can be trained to adapt this ability and, based on the training, recognize inconsistent data through machine learning - without having to use predefined rules as a basis.
A simple material with its dimensions in length, width and height serves as an illustrative example of the capabilities of such an AI system in the following. It is extremely complex or even impossible to describe in a defined rule how the relation of these three values should be, although everyone is aware that certain combinations of these values are valid in one's own product portfolio and others are not.
AI, and machine learning in particular, make it possible to check precisely this ratio, based on the product portfolio in question rather than on a specific set of rules.
ML systems create an algorithm that learns consistent as well as inconsistent model combinations from previous datasets and thus can validate new master records and issue warnings after some time of training.
Although the AI algorithm as well as the training of the models are not executed in the SAP MDG itself, for example on an R server, the validation during the re-entry of master records can be fully integrated into the MDG so that they can be executed via the MDG validation framework.
For the user, this makes it indistinguishable whether the data was validated by a classic business rule or an AI model.
S/4 transformation: AI can help
S/4 transformation projects are undoubtedly a big topic in SAP environments at the moment. In particular, those in which the introduction of a new SAP S/4 Hana system is accompanied by ERP harmonization.
A particular challenge in such harmonization and transformation projects is not only the standardization of processes but also the harmonization of data.
Often, those ERP systems have grown over decades, have been extended by different people and partners, and are therefore usually very heterogeneous in terms of both processes and data.
MDM teams are central to these initiatives and ensure that the systems' master data is merged and managed sustainably from then on. Machine learning can play an important role here as a supplement to the classic ETL (Extract, Transfer, Load) applications - for example, in mapping the data.
Instead of prescribing the exact mapping logics (data + rule = mapping), ML applications enable optimized mapping based on training data (data + training = mapping). The actual rules thereby move into the background.
The first ML-based standard software solutions already exist for the consolidation of customer and supplier data. ML approaches also help to harmonize values in the harmonization of master data. One example is the evaluation of transactional supplier data to determine optimal payment terms.
Chatbot: The new master data maintenance
MDM system users also expect master data applications to keep pace technologically. User experience and system performance are decisive for their acceptance. Particularly in terms of user experience, MDM applications have to stand comparison with private smartphone apps - and usually lose out.
Users today want master data to be maintained with a few clicks. Confrontation with a large number of master data fields is increasingly being critically questioned.
Personal assistants based on machine learning enable a whole new experience for the user. They mostly use speech-to-text and natural language understanding algorithms.
What normally means several clicks and entries for users can now be done via a simple voice command or chat entry. When questions arise, personal assistants offer help via buttons, selection fields and values, or record user feedback on new functions or performance.
Another advantage is that they allow master data maintenance using business language without having to be specific about the identification key (customizing value).
Companies that are pursuing the vision of AI-based master data maintenance with mostly small use cases are taking a pioneering position. Use cases are scarce, and AI also plays only a subordinate role at MDM trade events. Nevertheless, there is a constantly growing interest in the topic.