Proprietary or Open Source: No Either-Or
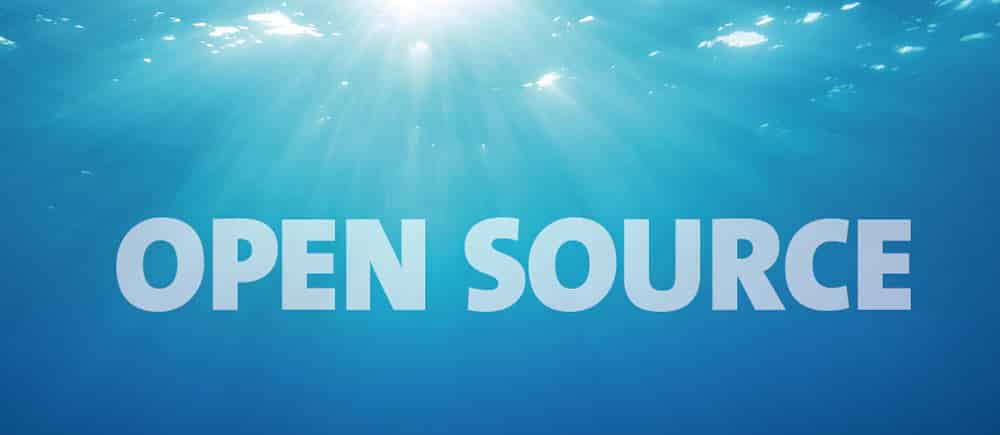
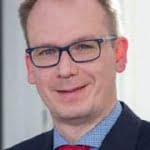
Proprietary or open, commercial or open source - this has long been a crucial question in IT. This discussion has not bypassed the evaluation of analytics software.
However, it is wrong to think of openness simply in terms of open source. Openness in the sense of a flexibly designable, agile IT infrastructure is more than just freely accessible source code. It goes much further.
Ultimately, openness is the characteristic I expect from software that I want to use to solve tricky and business-relevant analytics problems. In this sense, open means accessible. Unlocked. Ready to be used by anyone.
This openness should therefore apply to all analytical problems, users, competencies, company sizes, data volumes and IT environments. Only if every conceivable data source is integrated is it possible to include newer technological developments such as Hadoop or the cloud.
And only if external systems can also be functionally integrated will companies achieve the analytical agility they need in the future.
Against this background, the fundamental discussion about "commercial or open source" quickly vanishes into thin air. After all, apart from the question of the full costs of one solution or the other, which will continue to be the subject of considerable debate, it is primarily a matter of having the right functionalities, the right part of the application, the right piece of code in the right place at the right time.
Whether this comes from commercial software, is open source or a mixture of both is not so important to the company at that moment. In this respect, it is advisable for commercial software providers to integrate open source components into their own platform and enable seamless exchange with all common systems and formats.
And even more: In return, it must also be possible to integrate elements from commercial software into open source environments. For many, this looks like a cultural break.
And precisely: Wherever maximum agility is required, where innovation takes place, where analytics is the engine of digitization, the combination of open source and commercial analytical platforms will be implemented undogmatically and without an eye on labels.
Include manufacturer algorithms
In this interplay, commercial offerings will continue to form the starting point and analytical center of most architectures. They ensure the sustainable anchoring of an analytical culture in the company.
Durability (future-proof investments through compatibility and cloud-oriented architectures), deployment (transferring the analytical model to production), and governance (traceable and reproducible data processing procedures) are characteristics that such analytical ecosystems must possess.
What the future of coexistence and coexistence of open source and commercial software looks like? This is how it could go: An online service was essentially created with Python, open source that is.
If developers now want to provide users with better, i.e. more suitable, suggestions, they can use machine learning algorithms from a commercial software manufacturer to do so.
Something like this is already working today with the SAS Viya platform, which is geared to this new need for openness and dynamism. For this, we need an unprejudiced exchange between software companies and open source communities.
Always harping on the supposed weaknesses of the other is not a constructive solution. What is needed is to bundle the respective strengths. Customers have long since opted for this.